Role of AI in Cyber Security: A Comprehensive Guide
- -
- Time -
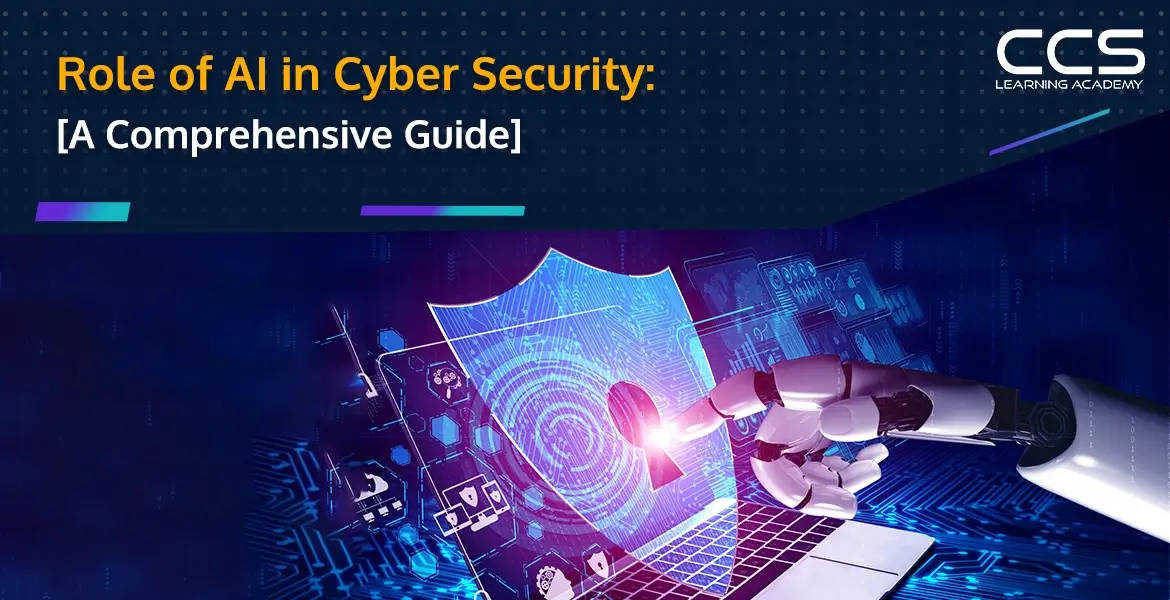
In recent years, the digital landscape has experienced an unprecedented surge in connectivity, driven by advancements in technology and the widespread adoption of online platforms. As our dependence on digital systems and networks has grown, so too has the threat landscape.
Cybersecurity has emerged as a critical concern, encompassing the practices and technologies designed to safeguard digital assets, information, and systems from unauthorized access, attacks, and damage.
As the complexity and scale of cyber threats continue to grow, traditional cybersecurity approaches face limitations in keeping pace with evolving attack methodologies. This is where the transformative role of AI in cybersecurity becomes apparent.
AI, a branch of computer science focusing on creating intelligent machines capable of performing tasks that typically require human intelligence, is increasingly leveraged to enhance cybersecurity measures.
- Types of Cyber Threats
- The Evolving Nature of Cyber Attacks
- Role of Artificial Intelligence (AI) in Enhancing Cybersecurity
- Key Components of AI in Cybersecurity
- Machine Learning and Its Applications in Threat Detection
- Applications of AI in Cybersecurity
- AI-Powered Security Analytics
- Steps for Organizations to Integrate AI into Their Cybersecurity Strategy
- Conclusion
- FAQs
Types of Cyber Threats
Cyber threats come in various forms, each presenting unique challenges to the security of digital systems.
These threats include:
- Malware: Software designed to harm or exploit systems, including viruses, worms, and ransomware.
- Phishing: Deceptive techniques trick individuals into revealing sensitive information, often through fraudulent emails or websites.
- Denial-of-Service (DoS) Attacks: Attempts to overwhelm a system or network, rendering it inaccessible to users.
- Insider Threats: Security risks from an organization, such as employees or contractors with malicious intent or unintentional negligence.
- Advanced Persistent Threats (APTs): Long-term targeted attacks by well-funded and organized adversaries seeking unauthorized access to sensitive information.
The Evolving Nature of Cyber Attacks
Cyberattacks are not static; they continually evolve in response to technological advancements and security measures. The rise of nation-state-sponsored attacks, the increasing sophistication of hacking techniques, and the expansion of the attack surface due to the Internet of Things (IoT) contribute to the dynamic nature of cyber threats.
Understanding cyber adversaries’ evolving tactics, techniques, and procedures is crucial for developing effective cybersecurity strategies.
The Understanding Cisco Cybersecurity Fundamentals (SECFND) course will improve your cybersecurity skills. Gain foundational knowledge in common security concepts, basic security techniques, and the fundamentals of applications, operating systems, and networking crucial for a Security Operations Center (SOC).
Equip yourself to detect, investigate, and respond effectively to diverse security events. Be a key player in your organization’s cybersecurity efforts. Enroll now and take the next step in advancing your career in cybersecurity.
Role of Artificial Intelligence (AI) in Enhancing Cybersecurity
Artificial Intelligence (AI) refers to the capability of machines to perform tasks that typically require human intelligence, such as learning, reasoning, problem-solving, and adapting to new situations.
In cybersecurity, AI is a transformative technology that augments traditional security measures by automating processes, analyzing vast datasets, and enhancing the ability to detect and respond to evolving cyber threats.
The role of AI in cybersecurity encompasses a range of techniques, including machine learning, natural language processing, and pattern recognition, to strengthen the resilience of digital systems against malicious activities. The goal is to enable computers to mimic human-like cognitive functions and proactively identify and mitigate potential risks in cybersecurity.
Key Components of AI in Cybersecurity
- Machine Learning Algorithms: At the core of AI in cybersecurity is machine learning, a subset of AI that enables systems to learn from data and improve their performance over time without explicit programming. Machine learning algorithms are crucial in detecting patterns, anomalies, and potential threats in vast datasets.
- Natural Language Processing (NLP): NLP allows machines to understand, interpret, and generate human-like language. In cybersecurity, NLP can analyze textual data, such as logs and threat intelligence reports, to extract meaningful insights and identify potential security issues.
- Predictive Analytics: AI utilizes predictive analytics to forecast future cyber threats based on historical data and ongoing trends. This proactive approach enables organizations to implement preventive measures before potential threats materialize.
- Automation and Orchestration: AI-driven automation streamlines routine cybersecurity tasks, allowing quicker response times and reducing the burden on human analysts. Security orchestration involves coordinating and automating various security processes to enhance overall efficiency.
Machine Learning and Its Applications in Threat Detection
Machine learning is a subset of AI that plays a pivotal role in improving the accuracy and speed of threat detection in cybersecurity.
Its applications include:
- Anomaly Detection Using AI Algorithms: Machine learning algorithms excel at identifying unusual patterns and behaviors within large datasets. In cybersecurity, these algorithms can detect anomalies that deviate from established norms, signaling potential security threats.
For example, anomalies in network traffic, user behavior, or system activities may indicate a compromised system or a cyberattack in progress.
- Behavioral Analysis for Identifying Unusual Patterns: Machine learning enables the creation of behavioral models for users, devices, and applications. AI can detect deviations that indicate malicious activities by continuously analyzing behavior patterns.
This approach is particularly effective in identifying insider threats or sophisticated attacks that evade traditional rule-based detection methods.
Applications of AI in Cybersecurity
Integrating the role of AI in cybersecurity applications significantly enhances the effectiveness of threat detection, incident response, and identity and access management.
These AI-driven approaches not only bolster security but also provide a more adaptive and responsive defense against the evolving tactics of cyber adversaries.
- Anomaly Detection Using AI Algorithms
Anomaly detection powered by AI algorithms is crucial to proactive threat detection. AI systems utilize machine learning algorithms to establish a baseline of normal behavior within a network or system.
By continuously analyzing patterns and activities, the AI can identify anomalies or deviations that may signify potential security threats. This method detects previously unseen attacks or subtle, sophisticated threats that traditional signature-based systems might miss.
- Algorithmic Learning: AI algorithms learn from historical data to understand normal behavior in a given environment.
- Real-Time Monitoring: Continuous monitoring of network and user activities in real-time allows for prompt detection of anomalies.
- Adaptability: AI systems can adapt to changes in the environment and update their understanding of normal behavior over time.
Example Scenario: An AI-powered anomaly detection system identifies unusual data access patterns within a corporate network, flagging a potential insider threat attempting unauthorized access to sensitive information.
- Behavioral Analysis for Identifying Unusual Patterns
Behavioral analysis, supported by machine learning, focuses on understanding and predicting the behavior of users, devices, and applications. By creating models of normal behavior, AI can identify deviations or anomalies that may indicate a security risk.
This approach is particularly valuable in detecting insider threats, where individuals within an organization may exhibit unusual behavior indicative of malicious intent.
- User Profiling: AI systems create profiles of typical behavior for users, devices, and applications.
- Dynamic Analysis: Continuous monitoring and analysis of behavior to detect deviations from established norms.
- Contextual Understanding: AI considers the context of activities to distinguish between normal and potentially malicious behavior.
Example Scenario: Behavioral analysis detects employees accessing sensitive files during non-working hours, deviating from their usual work patterns. The AI system raises an alert for further investigation.
- Automation of Incident Response Processes
AI-driven automation in incident response expedites the identification, containment, and resolution of security incidents.
Automated incident response processes leverage AI to execute predefined actions based on predefined rules and playbooks. This reduces response times and allows security teams to handle more incidents efficiently.
- Automated Playbooks: Predefined sequences of actions and responses to security incidents.
- Orchestration: Coordination of multiple automated processes for a comprehensive incident response.
- Workflow Integration: Seamless integration with existing security tools and systems.
Example Scenario: An AI-driven incident response system automatically isolates a compromised system from the network, changes access credentials, and notifies relevant stakeholders upon detecting a malware infection.
- AI-Driven Investigation and Remediation
AI contributes significantly to the investigation and remediation phases of incident response. Through advanced analytics and machine learning, AI aids in identifying the root cause of security incidents, understanding the tactics employed by attackers, and recommending effective remediation strategies.
- Threat Intelligence Integration: Incorporating threat intelligence feeds to enhance the accuracy of investigations.
- Forensic Analysis: AI assists in analyzing logs, network traffic, and other digital forensics data to reconstruct the timeline of events.
- Automated Remediation: AI recommends and, in some cases, automatically applies remediation measures to address security vulnerabilities.
Example Scenario: AI-driven investigation identifies a sophisticated phishing attack, analyzes the email headers and content, and automatically blocks similar malicious emails from reaching other users.
- Biometric Authentication and AI
AI enhances the reliability and security of biometric authentication methods, such as fingerprint, facial, and voice recognition.
By leveraging machine learning, AI systems can improve the accuracy of biometric authentication, making it more resistant to spoofing or impersonation.
- Biometric Pattern Learning: AI learns and refines the patterns associated with legitimate biometric data.
- Anti-Spoofing Measures: AI algorithms can detect and differentiate between genuine biometric data and spoofed attempts.
- Continuous Improvement: Ongoing learning allows AI systems to adapt to changes in biometric data over time.
Example Scenario: An AI-enhanced facial recognition system not only identifies individuals based on facial features but also adapts to changes in appearance due to factors like aging or changes in facial hair.
- Continuous Authentication Using Machine Learning
Continuous authentication involves ongoing user identity verification throughout a session rather than a one-time authentication process.
Machine learning contributes to continuous authentication by analyzing user behavior, device characteristics, and other contextual factors to ensure a consistent and secure user experience.
- User Behavior Analysis: Machine learning models establish a baseline of typical user behavior.
- Context-Aware Authentication: AI considers contextual factors, such as location and time of access, to enhance authentication accuracy.
- Risk-Based Authentication: Adaptive authentication based on the perceived risk level of the user’s activities.
Example Scenario: Continuous authentication using machine learning detects unusual behavior, such as multiple login attempts from different locations, triggering additional authentication measures to verify the user’s identity.
Take control of your cybersecurity career! Enroll in our CompTIA Cybersecurity Analyst (CySA+) course for a comprehensive approach to cybersecurity. These instructor-led classes are designed to empower professionals preparing for the CYSA+ (CS0-002) certification examination.
Gain the skills to confidently perform functions like IA and implement robust cybersecurity measures for your department.
AI-Powered Security Analytics
AI-powered security analytics, encompassing big data analytics, predictive analytics, and visualization tools, empowers cybersecurity professionals to gain actionable insights, predict and prevent potential threats, and comprehend the complexity of security data in an evolving digital landscape.
The synergy between AI and analytics provides a robust foundation for building adaptive and effective cybersecurity strategies.
- Big Data Analytics for Cybersecurity
In cybersecurity, the exponential growth of digital data generated by networks, systems, and applications has led to the need for advanced analytics to make sense of this vast information.
When combined with AI, big data analytics offers a powerful solution to analyze, correlate, and derive actionable insights from massive datasets in real time.
Data Collection and Aggregation
- Big data analytics in cybersecurity involves collecting and aggregating diverse datasets, including network logs, user activity logs, and threat intelligence feeds.
- AI algorithms process this data to identify patterns, anomalies, and trends indicating potential security threats.
Behavioral Analytics
- AI-powered big data analytics utilize machine learning to establish user, device, and system baseline behavior.
- By continuously analyzing behavior against established norms, anomalies indicative of potential threats can be detected.
Threat Hunting
- Security analysts can proactively leverage big data analytics and AI to hunt for potential threats within vast datasets.
- Machine learning algorithms assist in identifying subtle indicators of compromise that may go unnoticed through traditional methods.
Real-Time Analysis
- The combination of big data and AI allows for real-time analysis of security events, enabling swift detection and response to emerging threats.
- Rapid analysis is crucial in preventing or mitigating the impact of cyberattacks.
- Predictive Analytics in Identifying Potential Threats
Fueled by AI, predictive analytics forecasts future events based on historical data, patterns, and ongoing trends.
In cybersecurity, predictive analytics is crucial in proactively identifying potential threats before they manifest, allowing organizations to implement preemptive security measures.
Machine Learning Models
- Predictive analytics relies on machine learning models trained on historical data to recognize patterns associated with known threats.
- These models can then predict the likelihood of similar threats occurring in the future.
Behavioral Forecasting
- AI-driven predictive analytics extends beyond traditional threat signatures to forecast changes in user behavior, network traffic, and system activities.
- Organizations can anticipate and mitigate emerging threats by identifying deviations from expected behavior.
Risk Scoring
- Predictive analytics assigns risk scores to various entities, such as users or devices, based on their behavior and historical activities.
- High-risk scores trigger proactive security measures or additional scrutiny.
Dynamic Threat Intelligence Integration
- Predictive analytics integrates dynamic threat intelligence feeds to enhance the accuracy of predictions.
- The system continuously updates its models based on the latest threat intelligence data.
- Visualization Tools for Understanding Complex Security Data
Understanding complex security data is essential for effective decision-making and communication within cybersecurity teams.
AI-powered visualization tools transform intricate datasets into visually intuitive representations, facilitating the identification of patterns, trends, and anomalies.
Graphical Representations
- Visualization tools use graphs, charts, and heatmaps to represent complex relationships within security data.
- Graphical representations can reveal correlations between different security events and entities.
Interactive Dashboards
- AI-enhanced dashboards allow security analysts to interact with and manipulate visualized data dynamically.
- Analysts can drill down into specific details or zoom out for a high-level overview of the security landscape.
Behavioral Heatmaps
- Heatmaps visually depict user and system behavior patterns, making it easier to spot outliers or irregularities.
- AI algorithms contribute to the creation of heatmaps by identifying behavioral trends.
Threat Landscape Mapping
- Visualization tools map the overall threat landscape, highlighting the prevalence and distribution of various threats.
- This aids in prioritizing security efforts based on the most significant risks.
Steps for Organizations to Integrate AI into Their Cybersecurity Strategy
The role of AI in cybersecurity requires a strategic and well-planned approach.
Organizations can follow a series of steps to ensure the effective implementation of AI in their cybersecurity strategy:
- Assessment of Cybersecurity Needs
Conduct a thorough assessment of the organization’s cybersecurity needs, identifying key areas where AI can provide significant value.
Understand the existing cybersecurity infrastructure and determine where AI can complement or enhance current capabilities.
- Define Clear Objectives
Clearly define the objectives of the role of AI in cybersecurity strategy. Whether it’s improving threat detection, automating incident response, or enhancing identity and access management, having specific goals will guide the implementation process.
- Investment in AI Talent
Build a team of skilled AI professionals, including data scientists, machine learning engineers, and cybersecurity experts.
Train existing staff to ensure they understand AI’s capabilities and limitations in cybersecurity.
- Data Quality and Preparation
Ensure the quality and relevance of the data used to train and feed AI models. High-quality data is crucial for the effectiveness of AI algorithms.
Implement data governance practices to maintain sensitive information’s integrity, privacy, and security.
- Selecting Appropriate AI Technologies
Choose AI technologies that align with the specific cybersecurity needs of the organization.
Consider factors such as scalability, adaptability, and compatibility with existing security infrastructure.
- Pilot Projects and Testing
Begin with small-scale pilot projects to test the effectiveness of the role of AI in cybersecurity in real-world scenarios.
Use these pilot projects to fine-tune AI models, validate results, and identify any necessary adjustments.
- Integration with Existing Systems
Ensure seamless integration of AI-powered cybersecurity solutions with existing security tools and systems.
Develop APIs and interfaces allowing effective communication and coordination between AI components and other security measures.
- Continuous Monitoring and Improvement
Implement continuous monitoring of the role of AI in cybersecurity to identify and address issues promptly.
Establish a feedback loop for ongoing improvement, incorporating lessons learned from incidents and adapting AI models to evolving threats.
Conclusion
Integrating Artificial Intelligence (AI) into cybersecurity represents a transformative shift in how organizations defend against cyber threats. AI’s ability to process vast amounts of data, adapt to evolving attack methodologies, and automate complex tasks has elevated the effectiveness of cybersecurity measures.
From threat detection to incident response and identity management, the role of AI in cybersecurity empowers organizations to stay ahead of modern cyber threats’ dynamic and sophisticated nature.
Unlock your potential with CCS Learning Academy! Boost your skills and knowledge with our comprehensive courses. Whether diving into cybersecurity, exploring IT fundamentals, or advancing your career, CCS Learning Academy has the right path for you. Enroll now and embark on a journey of continuous learning and professional growth. Your future success starts here!
FAQs
Q1: What is the role of AI in cyber security?
Answer: AI plays a significant role in cyber security by enhancing threat detection, automating responses to cyber threats, and improving overall security systems. AI algorithms can analyze vast amounts of data to identify patterns and anomalies that may indicate a cyber threat, thus enabling faster and more effective responses.
Q2: How does AI improve threat detection in cyber security?
Answer: AI improves threat detection by using machine learning algorithms to analyze network traffic and identify unusual patterns that could signify a security breach. It can process large volumes of data much faster than humans, allowing for real-time threat detection and analysis.
Q3: Can AI be used to predict and prevent future cyber attacks?
Answer: Yes, AI can be used to predict potential cyber attacks by analyzing trends and patterns from past incidents. Machine learning models can be trained to recognize the precursors to attacks, enabling proactive measures to prevent them.
Q4: What are the benefits of using AI in cyber security?
Answer: The benefits of using AI in cyber security include enhanced detection of sophisticated threats, reduced response time to incidents, automation of security tasks, and the ability to analyze and process large datasets beyond human capability, leading to improved security posture.
Q5: Are there any challenges or limitations to using AI in cyber security?
Answer: Challenges include the risk of false positives, the need for continuous training of AI models, potential vulnerabilities in AI systems themselves, and the requirement for significant computational resources. Additionally, there is a need for skilled professionals to manage and interpret AI outputs.
Q6: How does AI help in responding to cyber security incidents?
Answer: AI aids in responding to cyber incidents by automating the initial steps such as segregating affected systems, blocking malicious IPs, and initiating security protocols. This rapid response can limit damage and provide valuable time for human analysts to intervene.
Q7: What is the role of machine learning in cyber security?
Answer: Machine learning, a subset of AI, plays a crucial role in cyber security by enabling systems to learn from past data, identify patterns, and make decisions with minimal human intervention. It’s used in threat detection, anomaly detection, and in developing predictive models.
Q8: Can AI replace human roles in cyber security?
Answer: While AI can automate and enhance many tasks in cyber security, it cannot entirely replace the need for human expertise. Human judgment is essential for interpreting AI findings, managing complex security strategies, and making decisions where context and nuanced understanding are required.
Q9: How is AI integrated into existing cyber security systems?
Answer: AI is integrated into existing cyber security systems through machine learning algorithms, natural language processing for threat intelligence, and AI-powered security tools like automated incident response systems and predictive analytics tools.
Q10: What future developments are expected in the use of AI in cyber security?
Answer: Future developments may include more advanced predictive analytics, enhanced automated response systems, improved AI algorithms for detecting zero-day vulnerabilities, and the integration of AI with other emerging technologies like blockchain for enhanced security solutions.
Q11: How can businesses start implementing AI in their cyber security strategies?
Answer: Businesses can start by adopting AI-powered security tools and software, investing in AI and machine learning training for their security teams, and partnering with AI-driven security service providers. It’s important to start with clear objectives and gradually integrate AI into existing security practices.
Q12: What ethical considerations are there when using AI in cyber security?
Answer: Ethical considerations include ensuring privacy and data protection, avoiding biased algorithms, maintaining transparency in AI-driven decisions, and considering the potential impact of automated actions on users and systems. It’s crucial to balance security enhancements with ethical responsibilities.